
Blog

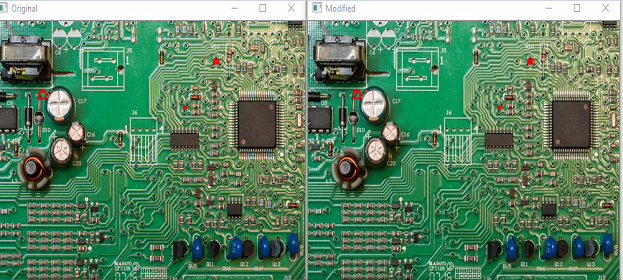
INTRODUCTION: Anomaly detection is a stage in data mining where data points, events, and/or observations veer off from a dataset’s ordinary behaviour. Anomalous data can critical incidents, for example, a specialized glitch, or possible chances, for example, an adjustment in customer behaviour. AI is dynamically being utilized to automate anomaly detection. NEED OF ANOMALY DETECTION: Current organizations are starting to comprehend the significance of interconnected activities to get the full image of their business. In addition, they have to react to quick moving changes in information instantly, particularly if there should be an occurrence of cyber security threats. Anomaly detection can be a key for illuminating such interruptions, as while distinguishing anomalies, annoyances of ordinary conduct show nearness of proposed or unintended attacks, defects, faults, and other threats. PROCESS OF ANOMALY DETECTION:-
- SUPERVISED MACHINE LEARNING FOR ANOMALY DETECTION:
This technique requires a labelled training set that contains both typical and anomalous samples for building the predictive model. Hypothetically, supervised methods are accepted to give a preferred identification rate over unsupervised techniques. Supervised neural systems, the definition of training model, support vector machine learning, k-nearest neighbours, Bayesian systems and decision trees. K-Nearest neighbour (k-NN) is one of the most conventional nonparametric techniques that are utilized in supervised learning for anomaly recognition.
It ascertains the approximate separations between various points on the input vectors and afterwards relegates the unlabelled highlight to the class of its K-nearest neighbours. The Bayesian system is another well-known model that can encode probabilistic connections among factors intrigue. This method is commonly utilized for anomaly identification in the mix with statistical plans. These supervised techniques have a few advantages, including the capacity of encoding interdependencies among factors and of predicting events, alongside the capacity to fuse both earlier knowledge and information.
- UNSUPERVISED MACHINE LEARNING FOR ANOMALY DETECTION
These methods don’t need training data. They depend on two essential presumptions. To begin with, they assume that the vast majority of the system associations are normal traffic and just a limited quantity of rate is abnormal. Second, they foresee that malicious traffic is statistically unique in relation to typical traffic.
Using these two assumptions, information gatherings of comparable cases that show up every now and again are thought to be normal traffic and those data groups that are inconsistent are viewed as malicious. The most widely recognized unsupervised calculations are self-organizing maps (SOM), K- means, C- means, expectation-maximization meta-algorithm (EM), adaptive resonance theory (ART), and one-class support vector machine. One famous method is a self-organizing map (SOM).
The primary goal of the SOM is to decrease the element of data visualization. Machine learning methods are currently accepting significant consideration among anomaly detection analysts to address the shortcomings of information base detection strategies. Anomaly detection can adequately help in getting the fraud, finding bizarre activity in large and complex Big Data sets. This can end up being helpful in territories, for example, banking security, natural sciences, medicine, and marketing, which are inclined to malicious exercises. With the machine, a learning association can strengthen search and increase the adequacy of their advanced business activities. APPLICATION OF ANOMALY DETECTION:-
- ANOMALY DETECTION IN HEALTH CARE:
Unfavourable occasions in healthcare and clinical blunders bring about a huge number of accidental deaths and more than 1,000,000 excess injuries every year. Anomaly detection in medicine is a significant assignment, particularly in the zone of radiation oncology where blunders are rare, however, can be incredibly hazardous, and even destructive To stay away from Medical blunders in radiation disease therapy, cautious attention should be made to guarantee precise usage of the expected treatment plan. Here we portray the work that brought about a significant valuable predictive analytics tool for automatic detection of catastrophic blunders in cancer radiotherapy, including significant protection for patient security.
Here a strategy for Dynamic Modelling and Prediction of Radiotherapy Treatment Deviations from Intended Plans (Smart Tool) has been formulated to naturally recognize and highlight likely mistakes in a radiotherapy treatment plan, in view of the information from a few thousand prostate cancer treatments that were utilized to build the model. Smart Tool decides whether the treatment boundaries are valid, against a formerly manufactured Predictive Model of a Medical Error (PMME). Smart Tool speaks with a radiotherapy treatment management system, checking all the treatment parameters in the background preceding execution, and after the human expert, QA is finished.
Any anomalous treatment parameters are recognized by utilizing an inventive intelligent algorithm in a totally automatic and unsupervised way, and it hails the operator by highlighting the suspect parameter(s) for human mediation. Besides, the framework is self-learning and continually developing, and the model is dynamically refreshed with the new treatment information.
- ANOMALY DETECTION IN THE MANUFACTURING SECTOR:-
Anomaly detection is getting progressively important to upgrade reliability and strength in the Industry 4.0 system. Here are various strategies for anomaly detection on manufacturing machines considering their fluctuation, both in operation and in wear conditions. We can see the type of accessible information, including any abnormality or not, is of significance for the algorithmic decision, talking about both statistical machine learning methods and control charts Finally to develop strategies for automatic anomaly detection, which get a review close to one on the information. The created strategies are structured not to depend on a persistent re-calibration and hand-tuning by the machine user, in this manner permitting their arrangement in an underway domain vigorously and effectively.
- ANOMALY DETECTION FOR AN E-COMMERCE PRICING SYSTEM:-
Online retailers execute an exceptionally huge number of price updates when contrasted with physical stores. Indeed, even a couple of misestimated things can have a noteworthy business effect and result in lost client trust. Early identification of anomalies in an automated real-time fashion is a significant piece of such an apprising system. The system here analyses the performance of different methodologies on a test set utilizing real-world retail information and completely sent this methodology into production. This system is able to find the most important anomalies with high precision.
CONCLUSION: Anomaly Detection has become one of the lethal weapons to detect fault detection, fraud detection and many other discrepancies in data. Several organizations are using Anomaly Detection for cost-effective and hazard-free business operations. We at Pixel Solutionz are providing end-to-end solutions for Anomaly Detection with AI. To avail of our service don’t hesitate to contact us.